Exploration and innovation are always costly activities. What makes it even more challenging is the fact that wrong design decision early, can results in wrong and very costly investments.
Heikki Immonen, Karelia University of Applied Sciences
The question you as an innovator should ask yourself, is how should you use your resources and time to find a good solution to the problem I am focusing on. Saving resources in the early stages can lead to massive cost overruns at later stages.
To help you make sense of what innovation strategy you should choose, this article will connect innovation process with the level and type of uncertainty you are facing.
Product life-cycle and level of uncertainty
Words like discovery, exploration, adventure and learning are of then linked with innovation. What all of these have in common is that they are words that describe how we related with the unknown. On the other hand, a mature business is all about focus and executing what we know and reaping the benefits.
To make sense of these too opposites, let’s look at how the so called product life-cycle, uncertainty and product development are related.
Product life-cycle
Product life-cycle or business life-cycle is graph that plots revenue (Y-axis) for each moment of time (X-axis). This curve has four distinctive stages: introduction, growth, maturity, and decline.
In the introduction phase the product is new, revenues are low, and the product experiences modifications based on initial feedback. When a scalable version of the product is discovered, revenue start to grow, until the maturity stage is reached. After steady high revenues, decline follows, as competition and shifting demand result in declining sales.
From the innovation process’ perspective we need to add one stage before the introduction stage. We can call it simply product-development or innovation stage. This is where the version of the product that is introduced to the markets at the introduction stage is being developed.
Innovation process can be seen as a process where uncertainty about what type of solution works, gets reduced
In order to better understand innovation, we can add another dimension to the graph. Let’s draw an uncertainty curve on top of the sales curve of the extended product life-cycle graph.
Uncertainty in this case can be understood as a probability that the current best guess, for what the product should be, will fail. In other words, it can also be seen as a measure of how much the current best guess of a successful product is different from the actual product that will be successful in the future. Yet another way to understand uncertainty is to think of it as the measure of errors or holes in the product developers’ understanding of the problem and reality.
Unlike revenues, uncertainty starts high and gets lower and lower until a very low point in the beginning of the growth stage. It then remains low through out maturity, but starts to grow with the decline of sales.
Early product-development stage defines bulk of total life-cycle costs
What organizations like NASA and other have discovered (Hirshorn et al. 2017), is that the bulk of product development costs are committed by the choices made early in the product development process. If these choices are made when uncertainty is still high, the choices are likely wrong and at later stages modifications are much more costly.
This is why early on in the process, much emphasis should be placed in getting the problem model, i.e., understanding of the problem as comprehensive and accurate as possible. It also means that key decision should be postponed until uncertainty is much reduced.
Innovation strategies balance investments with type and level of uncertainty
What innovation becomes, essentially, is balancing decision making and investments with the level of uncertainty you have. When uncertainty is high, avoid decision making and irreversible investments. Instead, put more resources to learning. When uncertainty is low, you don’t have to spend on learning and you can focus on speedily designing a solution and implementing it.
It’s like you the tourist vs. local taxi-driver in a big city. Taxi-driver can in seconds choose a route and start driving you to your target location. Without the taxi-driver, you have to spend time and energy getting a map, defining your location, studying and making sense of the map etc. before choosing a route. Even then you have to closely follow the map to make sure you have correctly positioned yourself on the map. Assuming of course, you had acquired a correct map and are holding it the right way 😊
Many aspects of reality are dominated by fundamental unremovable uncertainty
What makes things more complicated, is the fact that some domains of life and business are dominated by the type of uncertainty that is in a sense unremovable. It means that a perfect model of reality that could be used to develop a perfect solution can never be gained.
Domains that belong to this category are often called complex systems of which many human-related areas of life are good examples. In complex systems, actions cause counter actions and many types of feedback loops emerge, resulting in unpredictable outcomes. In situations like this, the strategy should not be anymore to gain perfect knowledge beforehand, but to emphasize adaptability to shifting circumstances, and protection from really bad outcomes (Taleb, 2012).
To get a better hold of what innovation strategy we should adopt given the level and type of uncertainty, let’s imagine a 2 by 2 uncertainty-complexity matrix. This matrix has four cells.
(NOTE: There is also another important dimension of uncertainty, which is related to harmful and catastrophic events. Before any intervention the risk of something rare, but really bad happening as a result of a new innovation should be understood well (Taleb, 2018).)
Low uncertainty – low complexity
First cell of the uncertainty matrix corresponds with low uncertainty-low complexity. This is when the developer has good understanding of the problem, and remaining uncertainty is not of the complex type. In this case you can adopt traditional design-implement strategy, where after a diagnostic stage a solution can be designed relatively quickly. Think of doctor or a taxi-driver who knows every street in the city.
High uncertainty – low complexity
Second cell is high uncertainty – low complexity. In this case the current problem model is very limited, but the uncertainty itself is not of the complex opaque type. Product developer can adopt a strategy where considerable time and effort is put to research and studies, to better understand the problem. After enough is learned a reliable working solution can be designed. Intelligent Fast Failure (IFF) is an example of this strategy. In IFF products are pushed until point of failure is reached, so that the failure mechanisms can then be understood and taken in to account (Camarda & de Weck, 2013).
Many technical problems not involving human components belong to this category. Adopting related innovation strategy would be like creating a good map by sending a drone to take aerial photographs of the city, and then selecting a route based on the information on the map.
Low uncertainty – high complexity
Third cell is low uncertainty – high complexity. In this case problem model is good, but there remains impenetrable complexity. A strategy for these types of situations is quickly implementing a simple best guess of a solution and then modifying and improving it based on feedback.
Navigating without a map, but with the help of a high and visible landmark at target destination, would correspond with this strategy. The overall goal is known, but expected unexpected obstacles have to be countered as they come.
So called agile product development strategies belong to this category. In architecture this would correspond with designing a building that can be easily and cheaply adapted to new and unexpected purposes (Brand, 1995).
High uncertainty – high complexity
Fourth cell corresponds with high uncertainty and high complexity. In this situation the developers have poor understanding of the problem and to make things more challenging, a lot of the uncertainty is of the complex type.
This type of situation requires a combination of two earlier strategies. On one hand you must study and research to acquire knowledge about areas of the problem where prior understanding is helpful. On the other hand, you must in an agile way test and adapt.
So called parallel testing strategies often allow you to move forward on both fronts. Testing and adaptation allow you to deal with complexity, while parallel testing allows you to discover rules and patterns not available for you via study of existing knowledge solely. This is like sending scouts to many directions to bring back knowledge of the surrounding city and possible routes.
The Innovation process
To sum up the lessons from above, in an archetypal innovation process we can recognize four distinctive sub-processes. There is the problem definition process, the learning or problem immersion process, ideation and evaluation process, and testing and implementing process.
This way you don’t rush in to implementing and investing an idea too early, but spend time in improving your understanding of the problem, and carefully make sure that you actually are really using all the knowledge you have acquired when designing solutions.
These processes are not linear, as each process can be a trigger of a re-do of an “earlier” sub-process. For example, evaluating your ideas using the knowledge you currently have might help you to realize you are having an important gap in your understanding of the problem. This will then trigger the learning and problem immersion process.
Developing ways to handle all of these four processes in an affordable and reliable manner can be seen as a key step in developing your and your organization’s innovation capability.
About this article
The writing of this article was supported by the INnoVations of REgional Sustainability: European UniversiTy Alliance project. https://www.invest-alliance.eu/ . This project is funded by the Erasmus+ Program.
The content of this article represents the views of the author only and is his sole responsibility. The European Commission and the Agency do not accept any responsibility for use that may be made of the information it contains.
References
Brand, S. (1995). How buildings learn: What happens after they’re built. Penguin.
Camarda, C. J., de Weck, O., & Do, S. (2013, June). Innovative Conceptual Engineering Design (ICED): Creativity and Innovation in a CDIO-Like Curriculum. In Proceedings of the 9th International CDIO Conference.
Hirshorn, S. R., Voss, L. D., & Bromley, L. K. (2017). Nasa systems engineering handbook
Taleb, N. N. (2012). Antifragile: how to live in a world we don’t understand. Random House
Taleb, N. N. (2018). Skin in the game: Hidden asymmetries in daily life. Random House.
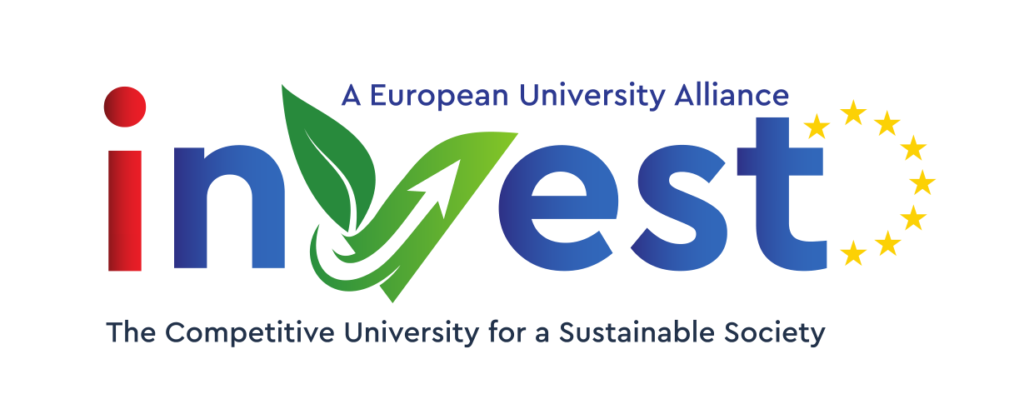
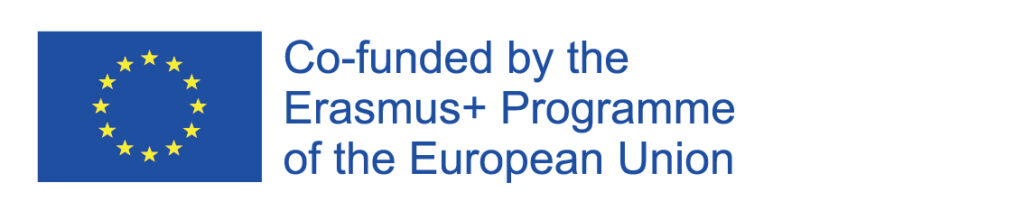